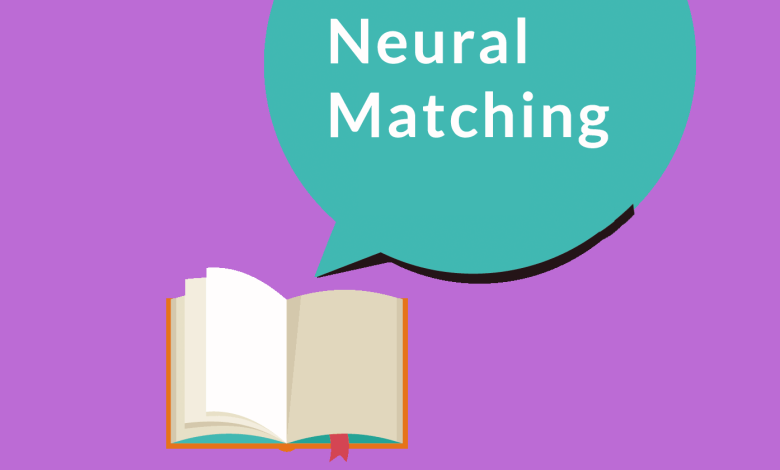
Google recently announced their implementation of a “neural matching” algorithm aimed at enhancing their understanding of concepts. Google’s Danny Sullivan noted that this algorithm is applied to 30% of search queries.
A newly published Google research paper demonstrates the potential of matching search queries to web pages using only the search query and the web pages themselves. Although this specific algorithm might not be currently in use or may be part of a suite of algorithms, it serves as an illustration of a potential application of a “neural matching” algorithm.
### Does Google Use Published Algorithms?
Google does not always deploy algorithms that appear in patents or research papers. However, some published algorithms are indeed part of Google’s search algorithms. It’s also important to note that Google rarely confirms whether a specific algorithm is in use.
### Google Discusses New AI Algorithm
In the past, Google has referred to its algorithms in general terms, such as the Panda and Penguin algorithms. Danny Sullivan appears to provide hints about the new “neural matching” algorithm. He described it as an AI method to better connect words to concepts.
Danny Sullivan also shared that people’s search behavior often differs from how solutions are written in content, suggesting the complexity in aligning search queries with web content.
### AI, Deep Learning, and Ranking Pages
Google’s AI Blog recently highlighted a research paper on Deep Relevance Ranking using Enhanced Document-Query Interactions. This research advances a deep neural network method focused on Document Relevance Ranking, also known as Ad-hoc Retrieval. While it is not confirmed that this is part of Google’s Neural Matching, it offers intriguing insights into similar methodologies.
The paper describes ad-hoc retrieval as ranking documents using only the query and document text. This method contrasts with standard information retrieval systems that harness text-based signals alongside network structure and user feedback.
Document Relevance Ranking stands out as a novel approach to ranking web pages, not relying on link signals.
### Does Google’s Document Relevance Ranking Not Use Links?
The algorithm discussed in Google’s AI blog does not directly utilize traditional ranking factors. Initially, these factors are still employed, followed by the Ad-hoc retrieval algorithm for re-ranking purposes. This process reveals that while traditional signals narrow down potential candidates, they do not solely dictate top-ten rankings. Traditional signals act as a filter for spam, identifying the most relevant documents. The subsequent algorithm focuses on matching against what Danny Sullivan termed “Super synonyms.” This partitioning distinguishes it from earlier algorithms like the Deep Relevance Matching Model (DRMM).
### What Does the Algorithm Actually Do?
The core function of this algorithm is matching a search query to a web page using only the query and the text of the page. Pages ranked by this method would not be promoted based solely on links or keywords, as the focus is on “relevance matching.”
### Content is More Important
Publishers should prioritize clear communication over inserting synonyms, as Google’s understanding of synonyms aims to comprehend the context and meaning of a page. Clarity and consistency in content are more valuable than keyword or synonym overuse. Google now focuses on understanding concepts, advancing beyond simple keywords to grasp how a page answers a search query.
The advancements in neural matching help generate search results not directly ranked by traditional factors like links or keywords. This underscores the importance of considering user intent and how well a page’s content assists users.
Understanding this approach helps publishers avoid ineffective methods like adding unnecessary synonyms. This new AI ranking illustrates the evolution in generating search results by emphasizing user intent and content efficacy.